
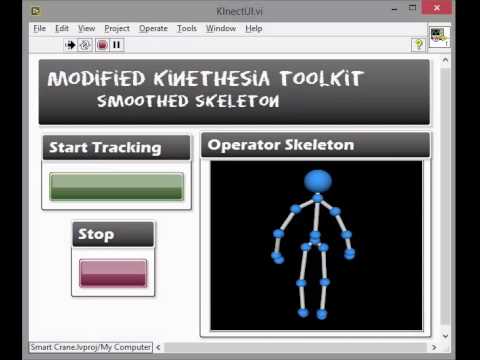
3dbaseline used a pretrained Stacked Hourglass network stacked_hourglass to generate 2D positions and then a simple fully connected network with residual blocks to. Sequences of keypoints for a single joint are our first level of abstraction.
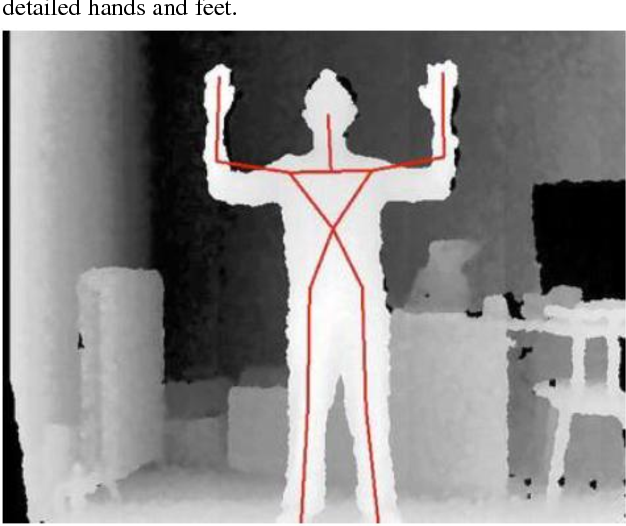

You will get really good Frames Per Second even on a mid-range GPU. Based on some search, I found out that Openpose runtime is independent from the number of the detected people whereas Alphapose’s performance depends on it and it increases linearly.
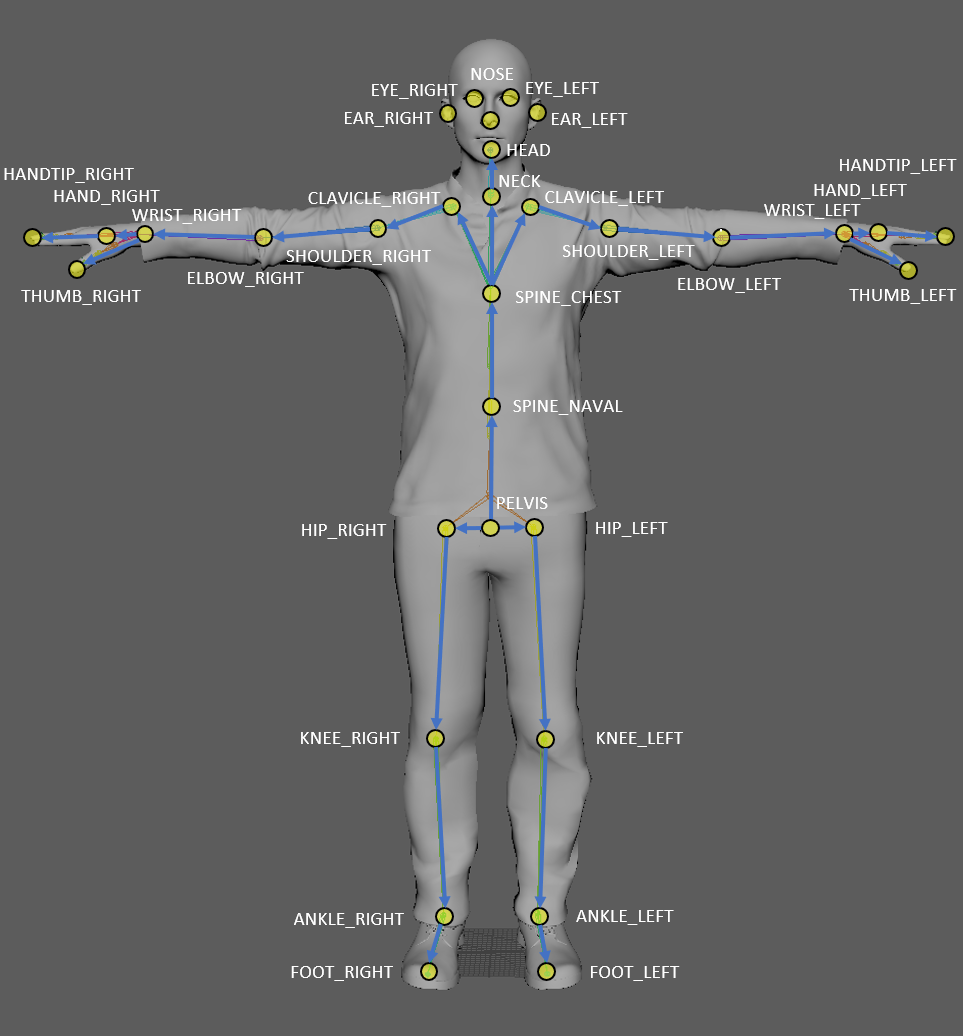
Wish You Were Here: Context-Aware Human Generation.If you want a complete solution in C++, then take a look at CMU's Openpose. Our method involves three subnetworks: the first generates the semantic map of the new person, given. Contains implementation of "Real-time 2D Multi-Person Pose Estimation on CPU: Lightweight OpenPose" paper. Personally, I come up that Alpha Pose is the best one, but I don't like the idea to integrate its PyTorch codebase to my TensorFlow pipeline. Whereas OpenPose allows up to 135 key points (including hands, face and feet), though we used 25 key points detection model (including foot key points). It's not a particularly exciting piece of programming, but I find it really useful as I don't have access to a computer which is powerful enough to run OpenPose, even in CPU mode, so the only way I can use OpenPose is to build it on a GPU-enabled Colab runtime and then run my. The mean difference and absolute difference in heel-strike and toe-off events was less than one video frame (40 ms sampling frequency of video recordings was 25 Hz) the greatest difference was two (80 ms) and three (120 ms) video frames for heel-strikes and toe. However, AlphaPose can only estimate body pose, and cannot detect key points in the hands. For more pretrained models, please refer to Model Zoo.
